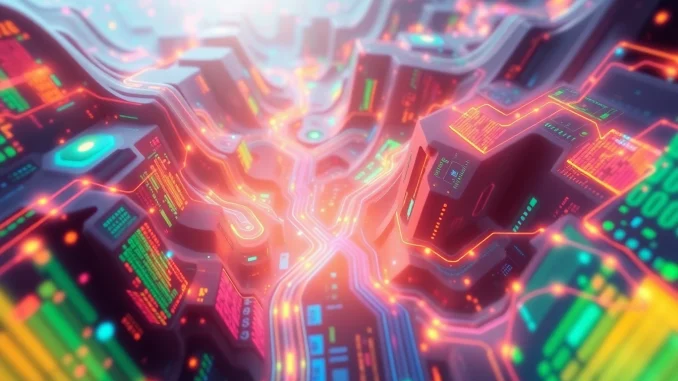
Understanding AI Sentiment Analysis
In the digital age, businesses generate and collect a vast amount of text data: reviews, social media comments, customer feedback, and more. Understanding the emotional tone behind this data has become a necessity for effective decision-making and strategic planning. This is where AI sentiment analysis plays a crucial role. By employing advanced algorithms and machine learning techniques, organizations can analyze sentiments expressed in this data, offering valuable insights into customer emotions, market trends, and overall brand perception.
What is Sentiment Analysis?
Sentiment analysis, often referred to as opinion mining, is the computational study of opinions, sentiments, and emotions conveyed in text. It aims to discern whether the sentiment expressed in a piece of text is positive, negative, or neutral. This process utilizes both natural language processing (NLP) and machine learning to evaluate text and classify the sentiment accordingly.
Importance of AI in Sentiment Analysis
The conventional methods of sentiment analysis were often labor-intensive and limited in scope. However, the advent of AI technologies has revolutionized this field. AI-powered sentiment analysis tools are capable of processing large volumes of text rapidly, providing businesses with real-time insights into customer opinions and emotions. The advantages of using AI include improved accuracy, scalability, and the ability to derive insights from unstructured data.
Common Applications Across Industries
Sentiment analysis is widely utilized across various sectors, including:
- Marketing and Brand Management: Companies analyze customer feedback to gauge sentiment towards their products and identify potential areas for improvement.
- Customer Service: AI sentiment analysis helps businesses quickly respond to negative sentiments expressed in customer inquiries, thereby enhancing customer satisfaction.
- Finance: Analysts use sentiment analysis to monitor public perceptions of market trends, aiding in predictive analytics.
- Healthcare: Providers analyze patient feedback and social media discussions to improve treatment plans and patient interactions.
Key Techniques in AI Sentiment Analysis
Natural Language Processing in Sentiment Analysis
Natural Language Processing (NLP) is a branch of artificial intelligence focused on the interaction between computers and humans through natural language. In sentiment analysis, NLP techniques are employed to parse text, identify relevant features, and extract meanings from words. NLP components such as tokenization, stemming, and lemmatization are crucial in preparing textual data for analysis.
Machine Learning Models for Accurate Predictions
Various machine learning models are adept at predicting sentiment, including:
- Naive Bayes: A probabilistic approach that assumes independence among features, commonly used for text classification.
- Support Vector Machines (SVM): Effective for high-dimensional spaces, SVM specializes in separating classes through hyperplanes.
- Deep Learning: Techniques such as recurrent neural networks (RNN) and long short-term memory networks (LSTM) have shown remarkable success in capturing the complexities of language.
Data Sources for Analyzing Sentiment
Sentiment analysis requires diverse datasets to train models and validate predictions. Common sources include:
- Social Media: Platforms like Twitter and Facebook provide vast amounts of unstructured data reflecting public sentiment.
- Customer Reviews: E-commerce platforms and review websites offer valuable insights into product sentiment.
- Surveys and Feedback Forms: Direct responses from customers are essential for understanding sentiment in structured formats.
Implementing AI Sentiment Analysis Tools
Choosing the Right AI Tools for Your Needs
When implementing AI sentiment analysis, selecting the right tools tailored for your specific requirements is vital. Considerations should include:
- Integration Capabilities: The tool should seamlessly integrate with existing systems and databases.
- Scalability: Ensure that the chosen tool can handle the volume of data you’re analyzing.
- Customization: Ability to adapt parameters and algorithms to fit unique dataset characteristics.
Step-by-Step Implementation Guide
Implementing an AI sentiment analysis system involves several steps:
- Define Objectives: Clearly outline what you aim to achieve through sentiment analysis.
- Data Collection: Gather diverse datasets from relevant sources.
- Data Preprocessing: Clean and prepare data for analysis using NLP techniques.
- Model Selection: Choose appropriate machine learning models based on your data characteristics.
- Training and Validation: Train your model on the dataset and validate its predictions using a test dataset.
- Deployment: Deploy the model to production and integrate it into your business workflow.
- Monitoring and Maintenance: Continuously monitor performance and retrain the model as required.
Integrating Sentiment Analysis into Business Workflows
For sentiment analysis to be effective, it must be woven into the fabric of business operations. This includes aligning sentiment analysis techniques with customer service protocols, marketing strategies, and product development initiatives. Regularly sharing insights from sentiment analysis across departments fosters a culture of data-driven decision-making.
Measuring Success in AI Sentiment Analysis
Key Performance Indicators (KPIs) to Track
Establishing KPIs helps measure the effectiveness of sentiment analysis initiatives. Consider tracking:
- Sentiment Accuracy: The percentage of accurately predicted sentiments compared to labeled data.
- Response Time: The time taken to respond to customer queries or complaints based on sentiment detection.
- Customer Satisfaction Scores: Monitoring changes in customer satisfaction before and after implementing sentiment analysis.
Evaluating Model Performance
Regular evaluation of model performance ensures continuous improvement. Metrics like precision, recall, and F1 score provide insights into the model’s predictive capabilities. A/B testing with real-world data helps gauge how well the model performs under different conditions and its adaptability to new data trends.
Case Studies of Successful Implementations
Several companies have successfully implemented AI-powered sentiment analysis to drive better business outcomes. For example:
- Netflix: Utilized sentiment analysis to understand viewer preferences and optimize content recommendations.
- Amazon: Analyzed customer reviews to enhance product offerings and customer service interventions.
- Spotify: Leveraged user feedback on social media to curate playlists and improve user engagement.
Future Trends in AI Sentiment Analysis
Advancements in Technology and Algorithms
The landscape of AI sentiment analysis is rapidly evolving, with ongoing advancements in technology. Trends such as enhanced NLP models, broader use of deep learning, and the integration of sentiment analysis with other AI functions (e.g., recommendation systems and chatbots) will likely enhance its effectiveness and applicability.
Ethical Considerations in Sentiment Analysis
As companies increasingly utilize sentiment analysis, ethical considerations such as data privacy, bias in machine learning models, and user consent are paramount. Organizations must ensure that their sentiment analysis techniques do not infringe upon user privacy or misinterpret sentiments due to algorithmic biases.
What to Expect in the Next Five Years
Looking ahead, we can anticipate that AI sentiment analysis will become more sophisticated, with increased accuracy and the ability to understand context better. Integration with AI-driven platforms and the growing importance of voice and visual sentiment analysis—looking beyond text to interpret nuances in human emotion—will shape the future of sentiment analysis.
Leave a Reply